Much ado about nothing: A comparison of missing data methods and software to fit incomplete data regression models
- PMID: 17401454
- PMCID: PMC1839993
- DOI: 10.1198/000313007X172556
Much ado about nothing: A comparison of missing data methods and software to fit incomplete data regression models
Abstract
Missing data are a recurring problem that can cause bias or lead to inefficient analyses. Development of statistical methods to address missingness have been actively pursued in recent years, including imputation, likelihood and weighting approaches. Each approach is more complicated when there are many patterns of missing values, or when both categorical and continuous random variables are involved. Implementations of routines to incorporate observations with incomplete variables in regression models are now widely available. We review these routines in the context of a motivating example from a large health services research dataset. While there are still limitations to the current implementations, and additional efforts are required of the analyst, it is feasible to incorporate partially observed values, and these methods should be utilized in practice.
Figures
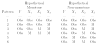
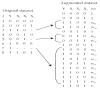
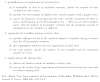
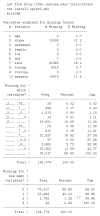
Similar articles
-
A nonparametric multiple imputation approach for missing categorical data.BMC Med Res Methodol. 2017 Jun 6;17(1):87. doi: 10.1186/s12874-017-0360-2. BMC Med Res Methodol. 2017. PMID: 28587662 Free PMC article.
-
Comparison of techniques for handling missing covariate data within prognostic modelling studies: a simulation study.BMC Med Res Methodol. 2010 Jan 19;10:7. doi: 10.1186/1471-2288-10-7. BMC Med Res Methodol. 2010. PMID: 20085642 Free PMC article.
-
Inverse Probability of Treatment Weighting and Confounder Missingness in Electronic Health Record-based Analyses: A Comparison of Approaches Using Plasmode Simulation.Epidemiology. 2023 Jul 1;34(4):520-530. doi: 10.1097/EDE.0000000000001618. Epub 2023 Apr 26. Epidemiology. 2023. PMID: 37155612 Free PMC article.
-
Review and evaluation of imputation methods for multivariate longitudinal data with mixed-type incomplete variables.Stat Med. 2022 Dec 30;41(30):5844-5876. doi: 10.1002/sim.9592. Epub 2022 Oct 11. Stat Med. 2022. PMID: 36220138 Free PMC article. Review.
-
Common Methods for Handling Missing Data in Marginal Structural Models: What Works and Why.Am J Epidemiol. 2021 Apr 6;190(4):663-672. doi: 10.1093/aje/kwaa225. Am J Epidemiol. 2021. PMID: 33057574 Free PMC article. Review.
Cited by
-
Single-case experimental designs: a systematic review of published research and current standards.Psychol Methods. 2012 Dec;17(4):510-50. doi: 10.1037/a0029312. Epub 2012 Jul 30. Psychol Methods. 2012. PMID: 22845874 Free PMC article. Review.
-
Assessing Alternative Imputation Strategies for Infrequently Missing Items on Multi-item Scales.Commun Stat Case Stud Data Anal Appl. 2022;8(4):682-713. doi: 10.1080/23737484.2022.2115430. Epub 2022 Sep 1. Commun Stat Case Stud Data Anal Appl. 2022. PMID: 36467970 Free PMC article.
-
Daratumumab-lenalidomide-dexamethasone vs standard-of-care regimens: Efficacy in transplant-ineligible untreated myeloma.Am J Hematol. 2020 Dec;95(12):1486-1494. doi: 10.1002/ajh.25963. Epub 2020 Sep 5. Am J Hematol. 2020. PMID: 32804408 Free PMC article. Clinical Trial.
-
ROBUST INFERENCE WHEN COMBINING INVERSE-PROBABILITY WEIGHTING AND MULTIPLE IMPUTATION TO ADDRESS MISSING DATA WITH APPLICATION TO AN ELECTRONIC HEALTH RECORDS-BASED STUDY OF BARIATRIC SURGERY.Ann Appl Stat. 2021 Mar;15(1):126-147. doi: 10.1214/20-aoas1386. Ann Appl Stat. 2021. PMID: 36245789 Free PMC article.
-
Missing data analysis using multiple imputation: getting to the heart of the matter.Circ Cardiovasc Qual Outcomes. 2010 Jan;3(1):98-105. doi: 10.1161/CIRCOUTCOMES.109.875658. Circ Cardiovasc Qual Outcomes. 2010. PMID: 20123676 Free PMC article. Review.
References
-
- Allison PD. Multiple imputation for missing data: a cautionary tale. Sociological Methods and Research. 2000;28:301–309.
-
- Allison PD. Missing data. SAGE University Papers; 2002.
-
- Allison PD. Imputation of categorical variables with PROC MI. 2005. [accessed July 30, 2006]. http://www2.sas.com/proceedings/sugi30/113-30.pdf.
-
- Barnard J, Meng XL. Applications of multiple imputation in medical studies: from AIDS to NHANES. Statistical Methods in Medical Research. 1999;8:17–36. - PubMed
-
- Bernaards CA, Belin TR, Schafer JL. Robustness of a multivariate normal approximation for imputation of incomplete binary data. Statistics in Medicine (In press) - PubMed
Grants and funding
LinkOut - more resources
Full Text Sources
Other Literature Sources