Modeling Actions of PubMed Users with N-Gram Language Models
- PMID: 19684883
- PMCID: PMC2727615
- DOI: 10.1007/s10791-008-9067-7
Modeling Actions of PubMed Users with N-Gram Language Models
Abstract
Transaction logs from online search engines are valuable for two reasons: First, they provide insight into human information-seeking behavior. Second, log data can be used to train user models, which can then be applied to improve retrieval systems. This article presents a study of logs from PubMed((R)), the public gateway to the MEDLINE((R)) database of bibliographic records from the medical and biomedical primary literature. Unlike most previous studies on general Web search, our work examines user activities with a highly-specialized search engine. We encode user actions as string sequences and model these sequences using n-gram language models. The models are evaluated in terms of perplexity and in a sequence prediction task. They help us better understand how PubMed users search for information and provide an enabler for improving users' search experience.
Figures
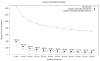
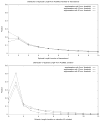
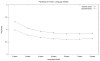
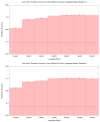
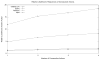
Similar articles
-
Understanding PubMed user search behavior through log analysis.Database (Oxford). 2009;2009:bap018. doi: 10.1093/database/bap018. Epub 2009 Nov 27. Database (Oxford). 2009. PMID: 20157491 Free PMC article.
-
Comparing image search behaviour in the ARRS GoldMiner search engine and a clinical PACS/RIS.J Biomed Inform. 2015 Aug;56:57-64. doi: 10.1016/j.jbi.2015.04.013. Epub 2015 May 19. J Biomed Inform. 2015. PMID: 26002820
-
Query log analysis of an electronic health record search engine.AMIA Annu Symp Proc. 2011;2011:915-24. Epub 2011 Oct 22. AMIA Annu Symp Proc. 2011. PMID: 22195150 Free PMC article.
-
Analyzing Medical Image Search Behavior: Semantics and Prediction of Query Results.J Digit Imaging. 2015 Oct;28(5):537-46. doi: 10.1007/s10278-015-9792-6. J Digit Imaging. 2015. PMID: 25810317 Free PMC article. Review.
-
Internet-based information-seeking behaviour amongst doctors and nurses: a short review of the literature.Health Info Libr J. 2010 Mar;27(1):2-10. doi: 10.1111/j.1471-1842.2010.00883.x. Health Info Libr J. 2010. PMID: 20402799 Review.
Cited by
-
Understanding PubMed user search behavior through log analysis.Database (Oxford). 2009;2009:bap018. doi: 10.1093/database/bap018. Epub 2009 Nov 27. Database (Oxford). 2009. PMID: 20157491 Free PMC article.
-
How user intelligence is improving PubMed.Nat Biotechnol. 2018 Oct 1. doi: 10.1038/nbt.4267. Online ahead of print. Nat Biotechnol. 2018. PMID: 30272675
-
Harnessing PubMed User Query Logs for Post Hoc Explanations of Recommended Similar Articles.ArXiv [Preprint]. 2024 Feb 5:arXiv:2402.03484v1. ArXiv. 2024. PMID: 38903741 Free PMC article. Preprint.
-
Effects of individual health topic familiarity on activity patterns during health information searches.JMIR Med Inform. 2015 Mar 17;3(1):e16. doi: 10.2196/medinform.3803. JMIR Med Inform. 2015. PMID: 25783222 Free PMC article.
-
Studying PubMed usages in the field for complex problem solving: Implications for tool design.J Am Soc Inf Sci Technol. 2013 May 1;64(5):874-92. doi: 10.1002/asi.22796. J Am Soc Inf Sci Technol. 2013. PMID: 24376375 Free PMC article.
References
-
- Agichtein Eugene, Brill Eric, Dumais Susan. Improving Web search ranking by incorporating user behavior information. Proceedings of the 29th Annual International ACM SIGIR Conference on Research and Development in Information Retrieval (SIGIR 2006); Seattle, Washington. 2006. pp. 19–26.
-
- Anick Peter. Using terminological feedback for Web search refinement—a log-based study. Proceedings of the 26th Annual International ACM SIGIR Conference on Research and Development in Information Retrieval (SIGIR 2003); Toronto, Canada. 2003. pp. 88–95.
-
- Beitzel Steven M, Jensen Eric C, Chowdhury Abdur, Grossman David, Frieder Ophir. Hourly analysis of a very large topically categorized Web query log. Proceedings of the 27th Annual International ACM SIGIR Conference on Research and Development in Information Retrieval (SIGIR 2004); Sheffield, United Kingdom. 2004. pp. 321–328.
-
- Broder Andrei. A taxonomy of Web search. SIGIR Forum. 2002;36(2):3–10.
-
- Cahan Mitchell Aaron. GRATEFUL MED: A tool for studying searching behavior. Medical Reference Services Quarterly. 1989;8(4):61–79. - PubMed
Grants and funding
LinkOut - more resources
Full Text Sources