Candidemia Risk Prediction (CanDETEC) Model for Patients With Malignancy: Model Development and Validation in a Single-Center Retrospective Study
- PMID: 34309570
- PMCID: PMC8367162
- DOI: 10.2196/24651
Candidemia Risk Prediction (CanDETEC) Model for Patients With Malignancy: Model Development and Validation in a Single-Center Retrospective Study
Erratum in
-
Correction: Candidemia Risk Prediction (CanDETEC) Model for Patients With Malignancy: Model Development and Validation in a Single-Center Retrospective Study.JMIR Med Inform. 2022 Jan 19;10(1):e36385. doi: 10.2196/36385. JMIR Med Inform. 2022. PMID: 35044928 Free PMC article.
Abstract
Background: Appropriate empirical treatment for candidemia is associated with reduced mortality; however, the timely diagnosis of candidemia in patients with sepsis remains poor.
Objective: We aimed to use machine learning algorithms to develop and validate a candidemia prediction model for patients with cancer.
Methods: We conducted a single-center retrospective study using the cancer registry of a tertiary academic hospital. Adult patients diagnosed with malignancies between January 2010 and December 2018 were included. Our study outcome was the prediction of candidemia events. A stratified undersampling method was used to extract control data for algorithm learning. Multiple models were developed-a combination of 4 variable groups and 5 algorithms (auto-machine learning, deep neural network, gradient boosting, logistic regression, and random forest). The model with the largest area under the receiver operating characteristic curve (AUROC) was selected as the Candida detection (CanDETEC) model after comparing its performance indexes with those of the Candida Score Model.
Results: From a total of 273,380 blood cultures from 186,404 registered patients with cancer, we extracted 501 records of candidemia events and 2000 records as control data. Performance among the different models varied (AUROC 0.771- 0.889), with all models demonstrating superior performance to that of the Candida Score (AUROC 0.677). The random forest model performed the best (AUROC 0.889, 95% CI 0.888-0.889); therefore, it was selected as the CanDETEC model.
Conclusions: The CanDETEC model predicted candidemia in patients with cancer with high discriminative power. This algorithm could be used for the timely diagnosis and appropriate empirical treatment of candidemia.
Keywords: candidemia; decision support; decision support systems, clinical; development; infection control; machine learning; model; precision medicine; prediction; risk; supervised machine learning; validation.
©Junsang Yoo, Si-Ho Kim, Sujeong Hur, Juhyung Ha, Kyungmin Huh, Won Chul Cha. Originally published in JMIR Medical Informatics (https://medinform.jmir.org), 26.07.2021.
Conflict of interest statement
Conflicts of Interest: None declared.
Figures
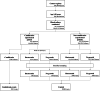
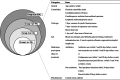
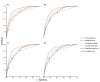
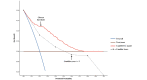
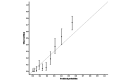
Similar articles
-
Prediction of candidemia with machine learning techniques: state of the art.Future Microbiol. 2024 Jul 2;19(10):931-940. doi: 10.2217/fmb-2023-0269. Epub 2024 May 20. Future Microbiol. 2024. PMID: 39072500 Review.
-
Development and Validation of Unplanned Extubation Prediction Models Using Intensive Care Unit Data: Retrospective, Comparative, Machine Learning Study.J Med Internet Res. 2021 Aug 11;23(8):e23508. doi: 10.2196/23508. J Med Internet Res. 2021. PMID: 34382940 Free PMC article.
-
Development and validation of a clinical prediction rule for candidemia in hospitalized patients with severe sepsis and septic shock.J Crit Care. 2015 Aug;30(4):715-20. doi: 10.1016/j.jcrc.2015.03.010. Epub 2015 Mar 14. J Crit Care. 2015. PMID: 25813550
-
Machine learning prediction of postoperative major adverse cardiovascular events in geriatric patients: a prospective cohort study.BMC Anesthesiol. 2022 Sep 10;22(1):284. doi: 10.1186/s12871-022-01827-x. BMC Anesthesiol. 2022. PMID: 36088288 Free PMC article.
-
Prediction of Cardiac Arrest in the Emergency Department Based on Machine Learning and Sequential Characteristics: Model Development and Retrospective Clinical Validation Study.JMIR Med Inform. 2020 Aug 4;8(8):e15932. doi: 10.2196/15932. JMIR Med Inform. 2020. PMID: 32749227 Free PMC article.
Cited by
-
Synthesis and Study of Antifungal Properties of New Cationic Beta-Glucan Derivatives.Pharmaceuticals (Basel). 2021 Aug 24;14(9):838. doi: 10.3390/ph14090838. Pharmaceuticals (Basel). 2021. PMID: 34577538 Free PMC article.
-
A machine learning model for early candidemia prediction in the intensive care unit: Clinical application.PLoS One. 2024 Sep 9;19(9):e0309748. doi: 10.1371/journal.pone.0309748. eCollection 2024. PLoS One. 2024. PMID: 39250466 Free PMC article.
-
Early diagnosis of candidemia with explainable machine learning on automatically extracted laboratory and microbiological data: results of the AUTO-CAND project.Ann Med. 2023;55(2):2285454. doi: 10.1080/07853890.2023.2285454. Epub 2023 Nov 27. Ann Med. 2023. PMID: 38010342 Free PMC article.
-
Prediction of candidemia with machine learning techniques: state of the art.Future Microbiol. 2024 Jul 2;19(10):931-940. doi: 10.2217/fmb-2023-0269. Epub 2024 May 20. Future Microbiol. 2024. PMID: 39072500 Review.
-
Multifaceted Evaluation of Antibiotic Therapy as a Factor Associated with Candidemia in Non-Neutropenic Patients.J Fungi (Basel). 2023 Feb 18;9(2):270. doi: 10.3390/jof9020270. J Fungi (Basel). 2023. PMID: 36836385 Free PMC article.
References
-
- Viscoli C, Girmenia C, Marinus A, Collette L, Martino P, Vandercam B, Doyen C, Lebeau B, Spence D, Krcmery V, De Pauw B, Meunier F. Candidemia in cancer patients: a prospective, multicenter surveillance study by the Invasive Fungal Infection Group (IFIG) of the European Organization for Research and Treatment of Cancer (EORTC) Clin Infect Dis. 1999 May;28(5):1071–9. doi: 10.1086/514731. - DOI - PubMed
-
- Tang H, Liu W, Lin H, Lai C. Epidemiology and prognostic factors of candidemia in cancer patients. PLoS One. 2014 Jun 5;9(6):e99103. doi: 10.1371/journal.pone.0099103. https://dx.plos.org/10.1371/journal.pone.0099103 PONE-D-14-02103 - DOI - DOI - PMC - PubMed
-
- Li D, Xia R, Zhang Q, Bai C, Li Z, Zhang P. Evaluation of candidemia in epidemiology and risk factors among cancer patients in a cancer center of China: an 8-year case-control study. BMC Infect Dis. 2017 Aug 03;17(1):536. doi: 10.1186/s12879-017-2636-x. https://bmcinfectdis.biomedcentral.com/articles/10.1186/s12879-017-2636-x 10.1186/s12879-017-2636-x - DOI - DOI - PMC - PubMed
LinkOut - more resources
Full Text Sources