Clinical Context-Aware Biomedical Text Summarization Using Deep Neural Network: Model Development and Validation
- PMID: 33095174
- PMCID: PMC7647812
- DOI: 10.2196/19810
Clinical Context-Aware Biomedical Text Summarization Using Deep Neural Network: Model Development and Validation
Abstract
Background: Automatic text summarization (ATS) enables users to retrieve meaningful evidence from big data of biomedical repositories to make complex clinical decisions. Deep neural and recurrent networks outperform traditional machine-learning techniques in areas of natural language processing and computer vision; however, they are yet to be explored in the ATS domain, particularly for medical text summarization.
Objective: Traditional approaches in ATS for biomedical text suffer from fundamental issues such as an inability to capture clinical context, quality of evidence, and purpose-driven selection of passages for the summary. We aimed to circumvent these limitations through achieving precise, succinct, and coherent information extraction from credible published biomedical resources, and to construct a simplified summary containing the most informative content that can offer a review particular to clinical needs.
Methods: In our proposed approach, we introduce a novel framework, termed Biomed-Summarizer, that provides quality-aware Patient/Problem, Intervention, Comparison, and Outcome (PICO)-based intelligent and context-enabled summarization of biomedical text. Biomed-Summarizer integrates the prognosis quality recognition model with a clinical context-aware model to locate text sequences in the body of a biomedical article for use in the final summary. First, we developed a deep neural network binary classifier for quality recognition to acquire scientifically sound studies and filter out others. Second, we developed a bidirectional long-short term memory recurrent neural network as a clinical context-aware classifier, which was trained on semantically enriched features generated using a word-embedding tokenizer for identification of meaningful sentences representing PICO text sequences. Third, we calculated the similarity between query and PICO text sequences using Jaccard similarity with semantic enrichments, where the semantic enrichments are obtained using medical ontologies. Last, we generated a representative summary from the high-scoring PICO sequences aggregated by study type, publication credibility, and freshness score.
Results: Evaluation of the prognosis quality recognition model using a large dataset of biomedical literature related to intracranial aneurysm showed an accuracy of 95.41% (2562/2686) in terms of recognizing quality articles. The clinical context-aware multiclass classifier outperformed the traditional machine-learning algorithms, including support vector machine, gradient boosted tree, linear regression, K-nearest neighbor, and naïve Bayes, by achieving 93% (16127/17341) accuracy for classifying five categories: aim, population, intervention, results, and outcome. The semantic similarity algorithm achieved a significant Pearson correlation coefficient of 0.61 (0-1 scale) on a well-known BIOSSES dataset (with 100 pair sentences) after semantic enrichment, representing an improvement of 8.9% over baseline Jaccard similarity. Finally, we found a highly positive correlation among the evaluations performed by three domain experts concerning different metrics, suggesting that the automated summarization is satisfactory.
Conclusions: By employing the proposed method Biomed-Summarizer, high accuracy in ATS was achieved, enabling seamless curation of research evidence from the biomedical literature to use for clinical decision-making.
Keywords: automatic text summarization; biomedical informatics; brain aneurysm; deep neural network; semantic similarity; word embedding.
©Muhammad Afzal, Fakhare Alam, Khalid Mahmood Malik, Ghaus M Malik. Originally published in the Journal of Medical Internet Research (http://www.jmir.org), 23.10.2020.
Conflict of interest statement
Conflicts of Interest: None declared.
Figures
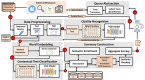
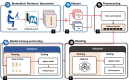
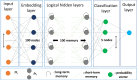
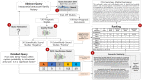
Similar articles
-
Quantifying the informativeness for biomedical literature summarization: An itemset mining method.Comput Methods Programs Biomed. 2017 Jul;146:77-89. doi: 10.1016/j.cmpb.2017.05.011. Epub 2017 May 27. Comput Methods Programs Biomed. 2017. PMID: 28688492
-
CERC: an interactive content extraction, recognition, and construction tool for clinical and biomedical text.BMC Med Inform Decis Mak. 2020 Dec 15;20(Suppl 14):306. doi: 10.1186/s12911-020-01330-8. BMC Med Inform Decis Mak. 2020. PMID: 33323109 Free PMC article.
-
Neural sentence embedding models for semantic similarity estimation in the biomedical domain.BMC Bioinformatics. 2019 Apr 11;20(1):178. doi: 10.1186/s12859-019-2789-2. BMC Bioinformatics. 2019. PMID: 30975071 Free PMC article.
-
Text summarization in the biomedical domain: a systematic review of recent research.J Biomed Inform. 2014 Dec;52:457-67. doi: 10.1016/j.jbi.2014.06.009. Epub 2014 Jul 10. J Biomed Inform. 2014. PMID: 25016293 Free PMC article. Review.
-
Neural network-based approaches for biomedical relation classification: A review.J Biomed Inform. 2019 Nov;99:103294. doi: 10.1016/j.jbi.2019.103294. Epub 2019 Sep 23. J Biomed Inform. 2019. PMID: 31557530 Review.
Cited by
-
The McMaster Health Information Research Unit: Over a Quarter-Century of Health Informatics Supporting Evidence-Based Medicine.J Med Internet Res. 2024 Jul 31;26:e58764. doi: 10.2196/58764. J Med Internet Res. 2024. PMID: 39083765 Free PMC article.
-
Initial Development of an Automated Platform for Assessing Trainee Performance on Case Presentations.ATS Sch. 2022 Sep 23;3(4):548-560. doi: 10.34197/ats-scholar.2022-0010OC. eCollection 2022 Dec. ATS Sch. 2022. PMID: 36726701 Free PMC article.
-
A systematic review of automatic text summarization for biomedical literature and EHRs.J Am Med Inform Assoc. 2021 Sep 18;28(10):2287-2297. doi: 10.1093/jamia/ocab143. J Am Med Inform Assoc. 2021. PMID: 34338801 Free PMC article.
-
Increasing Women's Knowledge about HPV Using BERT Text Summarization: An Online Randomized Study.Int J Environ Res Public Health. 2022 Jul 1;19(13):8100. doi: 10.3390/ijerph19138100. Int J Environ Res Public Health. 2022. PMID: 35805761 Free PMC article. Clinical Trial.
-
Comparing generative and extractive approaches to information extraction from abstracts describing randomized clinical trials.J Biomed Semantics. 2024 Apr 23;15(1):3. doi: 10.1186/s13326-024-00305-2. J Biomed Semantics. 2024. PMID: 38654304 Free PMC article.
References
-
- Nasr Azadani M, Ghadiri N, Davoodijam E. Graph-based biomedical text summarization: An itemset mining and sentence clustering approach. J Biomed Inform. 2018 Aug;84:42–58. doi: 10.1016/j.jbi.2018.06.005. https://linkinghub.elsevier.com/retrieve/pii/S1532-0464(18)30111-4 - DOI - PubMed
-
- Schulze F, Neves M. Entity-Supported Summarization of Biomedical Abstracts. The COLING 2016 Organizing Committee; Proceedings of the Fifth Workshop on Building and Evaluating Resources for Biomedical Text Mining (BioTxtM2016); December 2016; Osaka, Japan. pp. 40–49. https://www.aclweb.org/anthology/W16-5105/
-
- Alami N, Meknassi M, En-nahnahi N. Enhancing unsupervised neural networks based text summarization with word embedding and ensemble learning. Exp Syst App. 2019 Jun;123:195–211. doi: 10.1016/j.eswa.2019.01.037. - DOI
-
- Allahyari M, Pouriyeh S, Assefi M, Safaei S, D. E, B. J, Kochut K. Text Summarization Techniques: A Brief Survey. arXiv. 2017 Jul 28;:1–9. https://arxiv.org/abs/1707.02268v3
-
- Gambhir M, Gupta V. Recent automatic text summarization techniques: a survey. Artif Intell Rev. 2016 Mar 29;47(1):1–66. doi: 10.1007/s10462-016-9475-9. - DOI
Publication types
MeSH terms
LinkOut - more resources
Full Text Sources
Other Literature Sources