Classification of Clinically Useful Sentences in MEDLINE
- PMID: 26958301
- PMCID: PMC4765649
Classification of Clinically Useful Sentences in MEDLINE
Abstract
Objective: In a previous study, we investigated a sentence classification model that uses semantic features to extract clinically useful sentences from UpToDate, a synthesized clinical evidence resource. In the present study, we assess the generalizability of the sentence classifier to Medline abstracts.
Methods: We applied the classification model to an independent gold standard of high quality clinical studies from Medline. Then, the classifier trained on UpToDate sentences was optimized by re-retraining the classifier with Medline abstracts and adding a sentence location feature.
Results: The previous classifier yielded an F-measure of 58% on Medline versus 67% on UpToDate. Re-training the classifier on Medline improved F-measure to 68%; and to 76% (p<0.01) after adding the sentence location feature.
Conclusions: The classifier's model and input features generalized to Medline abstracts, but the classifier needed to be retrained on Medline to achieve equivalent performance. Sentence location provided additional contribution to the overall classification performance.
Figures
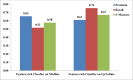
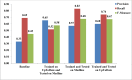
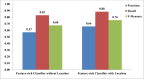
Similar articles
-
Classification of clinically useful sentences in clinical evidence resources.J Biomed Inform. 2016 Apr;60:14-22. doi: 10.1016/j.jbi.2016.01.003. Epub 2016 Jan 13. J Biomed Inform. 2016. PMID: 26774763 Free PMC article.
-
Categorization of sentence types in medical abstracts.AMIA Annu Symp Proc. 2003;2003:440-4. AMIA Annu Symp Proc. 2003. PMID: 14728211 Free PMC article.
-
LSAT: learning about alternative transcripts in MEDLINE.Bioinformatics. 2006 Apr 1;22(7):857-65. doi: 10.1093/bioinformatics/btk044. Epub 2006 Jan 12. Bioinformatics. 2006. PMID: 16410322
-
Semantic integration processes at different levels of syntactic hierarchy during sentence comprehension: an ERP study.Neuropsychologia. 2010 May;48(6):1551-62. doi: 10.1016/j.neuropsychologia.2010.02.001. Epub 2010 Feb 6. Neuropsychologia. 2010. PMID: 20138898
-
Large scale biomedical texts classification: a kNN and an ESA-based approaches.J Biomed Semantics. 2016 Jun 16;7:40. doi: 10.1186/s13326-016-0073-1. J Biomed Semantics. 2016. PMID: 27312781 Free PMC article.
Cited by
-
Classification of clinically useful sentences in clinical evidence resources.J Biomed Inform. 2016 Apr;60:14-22. doi: 10.1016/j.jbi.2016.01.003. Epub 2016 Jan 13. J Biomed Inform. 2016. PMID: 26774763 Free PMC article.
-
Automatic identification of recent high impact clinical articles in PubMed to support clinical decision making using time-agnostic features.J Biomed Inform. 2019 Jan;89:1-10. doi: 10.1016/j.jbi.2018.11.010. Epub 2018 Nov 22. J Biomed Inform. 2019. PMID: 30468912 Free PMC article.
-
Towards Evidence-based Precision Medicine: Extracting Population Information from Biomedical Text using Binary Classifiers and Syntactic Patterns.AMIA Jt Summits Transl Sci Proc. 2016 Jul 20;2016:203-12. eCollection 2016. AMIA Jt Summits Transl Sci Proc. 2016. PMID: 27570671 Free PMC article.
-
Formative evaluation of a patient-specific clinical knowledge summarization tool.Int J Med Inform. 2016 Feb;86:126-34. doi: 10.1016/j.ijmedinf.2015.11.006. Epub 2015 Nov 21. Int J Med Inform. 2016. PMID: 26612774 Free PMC article.
References
MeSH terms
Grants and funding
LinkOut - more resources
Full Text Sources