Enhancing text categorization with semantic-enriched representation and training data augmentation
- PMID: 16799127
- PMCID: PMC1561790
- DOI: 10.1197/jamia.M2051
Enhancing text categorization with semantic-enriched representation and training data augmentation
Abstract
Objective: Acquiring and representing biomedical knowledge is an increasingly important component of contemporary bioinformatics. A critical step of the process is to identify and retrieve relevant documents among the vast volume of modern biomedical literature efficiently. In the real world, many information retrieval tasks are difficult because of high data dimensionality and the lack of annotated examples to train a retrieval algorithm. Under such a scenario, the performance of information retrieval algorithms is often unsatisfactory, therefore improvements are needed.
Design: We studied two approaches that enhance the text categorization performance on sparse and high data dimensionality: (1) semantic-preserving dimension reduction by representing text with semantic-enriched features; and (2) augmenting training data with semi-supervised learning. A probabilistic topic model was applied to extract major semantic topics from a corpus of text of interest. The representation of documents was projected from the high-dimensional vocabulary space onto a semantic topic space with reduced dimensionality. A semi-supervised learning algorithm based on graph theory was applied to identify potential positive training cases, which were further used to augment training data. The effects of data transformation and augmentation on text categorization by support vector machine (SVM) were evaluated.
Results and conclusion: Semantic-enriched data transformation and the pseudo-positive-cases augmented training data enhance the efficiency and performance of text categorization by SVM.
Figures
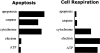
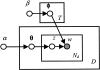
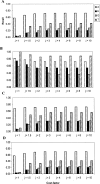
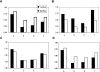
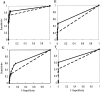
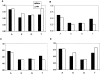
Similar articles
-
Text categorization of biomedical data sets using graph kernels and a controlled vocabulary.IEEE/ACM Trans Comput Biol Bioinform. 2013 Sep-Oct;10(5):1211-7. doi: 10.1109/TCBB.2013.16. IEEE/ACM Trans Comput Biol Bioinform. 2013. PMID: 24384709
-
Enhancing biomedical text summarization using semantic relation extraction.PLoS One. 2011;6(8):e23862. doi: 10.1371/journal.pone.0023862. Epub 2011 Aug 26. PLoS One. 2011. PMID: 21887336 Free PMC article.
-
Representing clinical questions by semantic type for better classification.AMIA Annu Symp Proc. 2006;2006:987. AMIA Annu Symp Proc. 2006. PMID: 17238606 Free PMC article.
-
A critical review of PASBio's argument structures for biomedical verbs.BMC Bioinformatics. 2006 Nov 24;7 Suppl 3(Suppl 3):S5. doi: 10.1186/1471-2105-7-S3-S5. BMC Bioinformatics. 2006. PMID: 17134478 Free PMC article. Review.
-
Hierarchical semantic structures for medical NLP.Stud Health Technol Inform. 2013;192:1194. Stud Health Technol Inform. 2013. PMID: 23920968 Review.
Cited by
-
A Kernel Theory of Modern Data Augmentation.Proc Mach Learn Res. 2019 Jun;97:1528-1537. Proc Mach Learn Res. 2019. PMID: 31777848 Free PMC article.
-
Developing Embedded Taxonomy and Mining Patients' Interests From Web-Based Physician Reviews: Mixed-Methods Approach.J Med Internet Res. 2018 Aug 16;20(8):e254. doi: 10.2196/jmir.8868. J Med Internet Res. 2018. PMID: 30115610 Free PMC article. Review.
-
Learning to Compose Domain-Specific Transformations for Data Augmentation.Adv Neural Inf Process Syst. 2017 Dec;30:3239-3249. Adv Neural Inf Process Syst. 2017. PMID: 29375240 Free PMC article.
-
Mapping annotations with textual evidence using an scLDA model.AMIA Annu Symp Proc. 2011;2011:834-42. Epub 2011 Oct 22. AMIA Annu Symp Proc. 2011. PMID: 22195141 Free PMC article.
-
Artificial Intelligence in Medicine: Chances and Challenges for Wide Clinical Adoption.Visc Med. 2020 Dec;36(6):443-449. doi: 10.1159/000511930. Epub 2020 Oct 12. Visc Med. 2020. PMID: 33442551 Free PMC article. Review.
References
-
- Hersh WR, Bhuptiraju RT, Ross L, Johnson P, Cohen AM, Kreamer DF. TREC 2004 genomics track overview. 2004. Paper presented at: Text Retrieval Conference (TREC) 2004.
-
- Hersh W, Bhupatiraju R. TREC genomics track overview. 2003. Paper presented at: Twelfth Text Retrieval Conference - TREC 2003.
Publication types
MeSH terms
Grants and funding
LinkOut - more resources
Full Text Sources
Other Literature Sources