Heterogeneity estimation in meta-analysis of standardized mean differences when the distribution of random effects departs from normal: A Monte Carlo simulation study
- PMID: 36650428
- PMCID: PMC9843903
- DOI: 10.1186/s12874-022-01809-0
Heterogeneity estimation in meta-analysis of standardized mean differences when the distribution of random effects departs from normal: A Monte Carlo simulation study
Abstract
Background: Advantages of meta-analysis depend on the assumptions underlying the statistical procedures used being met. One of the main assumptions that is usually taken for granted is the normality underlying the population of true effects in a random-effects model, even though the available evidence suggests that this assumption is often not met. This paper examines how 21 frequentist and 24 Bayesian methods, including several novel procedures, for computing a point estimate of the heterogeneity parameter ([Formula: see text]) perform when the distribution of random effects departs from normality compared to normal scenarios in meta-analysis of standardized mean differences.
Methods: A Monte Carlo simulation was carried out using the R software, generating data for meta-analyses using the standardized mean difference. The simulation factors were the number and average sample size of primary studies, the amount of heterogeneity, as well as the shape of the random-effects distribution. The point estimators were compared in terms of absolute bias and variance, although results regarding mean squared error were also discussed.
Results: Although not all the estimators were affected to the same extent, there was a general tendency to obtain lower and more variable [Formula: see text] estimates as the random-effects distribution departed from normality. However, the estimators ranking in terms of their absolute bias and variance did not change: Those estimators that obtained lower bias also showed greater variance. Finally, a large number and sample size of primary studies acted as a bias-protective factor against a lack of normality for several procedures, whereas only a high number of studies was a variance-protective factor for most of the estimators analyzed.
Conclusions: Although the estimation and inference of the combined effect have proven to be sufficiently robust, our work highlights the role that the deviation from normality may be playing in the meta-analytic conclusions from the simulation results and the numerical examples included in this work. With the aim to exercise caution in the interpretation of the results obtained from random-effects models, the tau2() R function is made available for obtaining the range of [Formula: see text] values computed from the 45 estimators analyzed in this work, as well as to assess how the pooled effect, its confidence and prediction intervals vary according to the estimator chosen.
Keywords: Between-study variance; Heterogeneity; Meta-analysis; Non-normality; Random effects; Simulation study.
© 2023. The Author(s).
Conflict of interest statement
The authors declare that they have no competing interests.
Figures
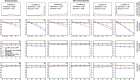
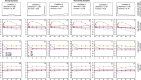
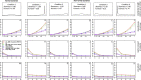
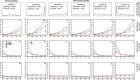
Similar articles
-
Folic acid supplementation and malaria susceptibility and severity among people taking antifolate antimalarial drugs in endemic areas.Cochrane Database Syst Rev. 2022 Feb 1;2(2022):CD014217. doi: 10.1002/14651858.CD014217. Cochrane Database Syst Rev. 2022. PMID: 36321557 Free PMC article.
-
Estimation of an overall standardized mean difference in random-effects meta-analysis if the distribution of random effects departs from normal.Res Synth Methods. 2018 Sep;9(3):489-503. doi: 10.1002/jrsm.1312. Epub 2018 Jul 30. Res Synth Methods. 2018. PMID: 29989344
-
A comparison of heterogeneity variance estimators in combining results of studies.Stat Med. 2007 Apr 30;26(9):1964-81. doi: 10.1002/sim.2688. Stat Med. 2007. PMID: 16955539
-
Applications of Monte Carlo Simulation in Modelling of Biochemical Processes.In: Mode CJ, editor. Applications of Monte Carlo Methods in Biology, Medicine and Other Fields of Science [Internet]. Rijeka (HR): InTech; 2011 Feb 28. Chapter 4. In: Mode CJ, editor. Applications of Monte Carlo Methods in Biology, Medicine and Other Fields of Science [Internet]. Rijeka (HR): InTech; 2011 Feb 28. Chapter 4. PMID: 28045483 Free Books & Documents. Review.
-
Improving Methods for Discrete Choice Experiments to Measure Patient Preferences [Internet].Washington (DC): Patient-Centered Outcomes Research Institute (PCORI); 2021 Mar. Washington (DC): Patient-Centered Outcomes Research Institute (PCORI); 2021 Mar. PMID: 38386769 Free Books & Documents. Review.
Cited by
-
A Meta-Analytical Way of Systematizing the Use of Hyaluronan Gels for the Relief of Osteoarthritis, Compared with New Treatment Alternatives.Gels. 2024 Jul 20;10(7):481. doi: 10.3390/gels10070481. Gels. 2024. PMID: 39057505 Free PMC article.
-
Comparing robotic and open partial nephrectomy under the prism of surgical precision: a meta-analysis of the average blood loss rate as a novel variable.J Robot Surg. 2024 Aug 7;18(1):313. doi: 10.1007/s11701-024-02060-z. J Robot Surg. 2024. PMID: 39112829 Free PMC article. Review.
-
The normality assumption on between-study random effects was questionable in a considerable number of Cochrane meta-analyses.BMC Med. 2023 Mar 29;21(1):112. doi: 10.1186/s12916-023-02823-9. BMC Med. 2023. PMID: 36978059 Free PMC article.
-
Ways to improve the methodology of meta-analysis in sports and exercise medicine: what do we often see in peer review?BMJ Open Sport Exerc Med. 2024 Sep 28;10(3):e002256. doi: 10.1136/bmjsem-2024-002256. eCollection 2024. BMJ Open Sport Exerc Med. 2024. PMID: 39351124 Free PMC article. No abstract available.
References
-
- Rubio-Aparicio M, López-López JA, Sánchez-Meca J, Marín-Martínez F, Viechtbauer W, Van den Noortgate W. Estimation of an overall standardized mean difference in random-effects meta-analysis if the distribution of random effects departs from normal. Res Synth Methods. 2018;9(3):489–503. doi: 10.1002/jrsm.1312. - DOI - PubMed
Publication types
MeSH terms
Grants and funding
LinkOut - more resources
Full Text Sources
Miscellaneous