Comparison of exclusion, imputation and modelling of missing binary outcome data in frequentist network meta-analysis
- PMID: 32111167
- PMCID: PMC7049189
- DOI: 10.1186/s12874-020-00929-9
Comparison of exclusion, imputation and modelling of missing binary outcome data in frequentist network meta-analysis
Abstract
Background: Missing participant outcome data (MOD) are ubiquitous in systematic reviews with network meta-analysis (NMA) as they invade from the inclusion of clinical trials with reported participant losses. There are available strategies to address aggregate MOD, and in particular binary MOD, while considering the missing at random (MAR) assumption as a starting point. Little is known about their performance though regarding the meta-analytic parameters of a random-effects model for aggregate binary outcome data as obtained from trial-reports (i.e. the number of events and number of MOD out of the total randomised per arm).
Methods: We used four strategies to handle binary MOD under MAR and we classified these strategies to those modelling versus excluding/imputing MOD and to those accounting for versus ignoring uncertainty about MAR. We investigated the performance of these strategies in terms of core NMA estimates by performing both an empirical and simulation study using random-effects NMA based on electrical network theory. We used Bland-Altman plots to illustrate the agreement between the compared strategies, and we considered the mean bias, coverage probability and width of the confidence interval to be the frequentist measures of performance.
Results: Modelling MOD under MAR agreed with exclusion and imputation under MAR in terms of estimated log odds ratios and inconsistency factor, whereas accountability or not of the uncertainty regarding MOD affected intervention hierarchy and precision around the NMA estimates: strategies that ignore uncertainty about MOD led to more precise NMA estimates, and increased between-trial variance. All strategies showed good performance for low MOD (<5%), consistent evidence and low between-trial variance, whereas performance was compromised for large informative MOD (> 20%), inconsistent evidence and substantial between-trial variance, especially for strategies that ignore uncertainty due to MOD.
Conclusions: The analysts should avoid applying strategies that manipulate MOD before analysis (i.e. exclusion and imputation) as they implicate the inferences negatively. Modelling MOD, on the other hand, via a pattern-mixture model to propagate the uncertainty about MAR assumption constitutes both conceptually and statistically proper strategy to address MOD in a systematic review.
Keywords: Imputation; Missing outcome data; Network meta-analysis; Pattern-mixture model; Systematic review.
Conflict of interest statement
The authors declare that they have no competing interests.
Figures
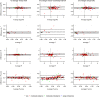
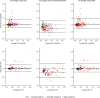
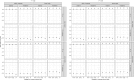
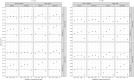
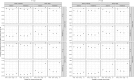
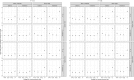
Similar articles
-
Pattern-mixture model in network meta-analysis of binary missing outcome data: one-stage or two-stage approach?BMC Med Res Methodol. 2021 Jan 7;21(1):12. doi: 10.1186/s12874-020-01205-6. BMC Med Res Methodol. 2021. PMID: 33413138 Free PMC article.
-
An empirical comparison of Bayesian modelling strategies for missing binary outcome data in network meta-analysis.BMC Med Res Methodol. 2019 Apr 24;19(1):86. doi: 10.1186/s12874-019-0731-y. BMC Med Res Methodol. 2019. PMID: 31018836 Free PMC article.
-
Modeling missing binary outcome data while preserving transitivity assumption yielded more credible network meta-analysis results.J Clin Epidemiol. 2019 Jan;105:19-26. doi: 10.1016/j.jclinepi.2018.09.002. Epub 2018 Sep 14. J Clin Epidemiol. 2019. PMID: 30223064
-
Dealing with missing outcome data in meta-analysis.Res Synth Methods. 2020 Jan;11(1):2-13. doi: 10.1002/jrsm.1349. Epub 2019 Jun 9. Res Synth Methods. 2020. PMID: 30991455 Free PMC article. Review.
-
Systematic review of interventions for treating or preventing antipsychotic-induced tardive dyskinesia.Health Technol Assess. 2017 Aug;21(43):1-218. doi: 10.3310/hta21430. Health Technol Assess. 2017. PMID: 28812541 Free PMC article. Review.
Cited by
-
Pattern-mixture model in network meta-analysis of binary missing outcome data: one-stage or two-stage approach?BMC Med Res Methodol. 2021 Jan 7;21(1):12. doi: 10.1186/s12874-020-01205-6. BMC Med Res Methodol. 2021. PMID: 33413138 Free PMC article.
-
Continuous(ly) missing outcome data in network meta-analysis: A one-stage pattern-mixture model approach.Stat Methods Med Res. 2021 Apr;30(4):958-975. doi: 10.1177/0962280220983544. Epub 2021 Jan 6. Stat Methods Med Res. 2021. PMID: 33406990 Free PMC article.
References
-
- Akl EA, Carrasco-Labra A, Brignardello-Petersen R, Neumann I, Johnston BC, Sun X, et al. Reporting, handling and assessing the risk of bias associated with missing participant data in systematic reviews: a methodological survey. BMJ Open. 2015;5:e009368. doi: 10.1136/bmjopen-2015-009368. - DOI - PMC - PubMed
Publication types
MeSH terms
LinkOut - more resources
Full Text Sources