Structure-based modeling of critical micelle concentration (CMC) of anionic surfactants in brine using intelligent methods
- PMID: 37591920
- PMCID: PMC10435457
- DOI: 10.1038/s41598-023-40466-1
Structure-based modeling of critical micelle concentration (CMC) of anionic surfactants in brine using intelligent methods
Abstract
Critical micelle concentration (CMC) is one of the main physico-chemical properties of surface-active agents, also known as surfactants, with diverse theoretical and industrial applications. It is influenced by basic parameters such as temperature, pH, salinity, and the chemical structure of surfactants. Most studies have only estimated CMC at fixed conditions based on the surfactant's chemical parameters. In the present study, we aimed to develop a set of novel and applicable models for estimating CMC of well-known anionic surfactants by considering both the molecular properties of surfactants and basic affecting factors such as salinity, pH, and temperature as modeling parameters. We employed the quantitative-structural property relationship technique to employ the molecular parameters of surfactant ions. We collected 488 CMC values from literature for 111 sodium-based anionic surfactants, including sulfate types, sulfonate, benzene sulfonate, sulfosuccinate, and polyoxyethylene sulfate. We computed 1410 optimized molecular descriptors for each surfactant using Dragon software to be utilized in the modelling processes. The enhanced replacement method was used for selecting the most effective descriptors for the CMC. A multivariate linear model and two non-linear models are the outputs of the present study. The non-linear models were produced using two robust machine learning approaches, stochastic gradient boosting (SGB) trees and genetic programming (GP). Statistical assessment showed highly applicable and acceptable accuracy of the newly developed models (RSGB2 = 0.999395 and RGP2 = 0.954946). The ultimate results showed the superiority and greater ability of the SGB method for making confident predictions.
© 2023. Springer Nature Limited.
Conflict of interest statement
The authors certify that they have NO conflict over any financial interest or non-financial interest in the subject matter or materials discussed in this manuscript.
Figures
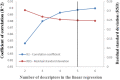
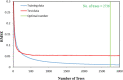
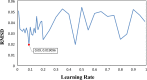
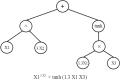
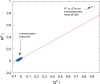
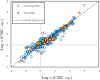
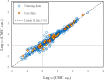
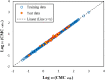
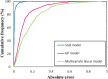
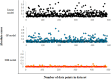
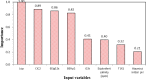
Similar articles
-
CMC prediction for ionic surfactants in pure water and aqueous salt solutions based solely on tabulated molecular parameters.J Colloid Interface Sci. 2017 Sep 1;501:142-149. doi: 10.1016/j.jcis.2017.04.046. Epub 2017 Apr 18. J Colloid Interface Sci. 2017. PMID: 28448834
-
QSPR study of critical micelle concentration of anionic surfactants using computational molecular descriptors.J Chem Inf Model. 2007 May-Jun;47(3):782-93. doi: 10.1021/ci600462d. Epub 2007 May 12. J Chem Inf Model. 2007. PMID: 17497845
-
Quantifying the hydrophobic effect. 2. A computer simulation-molecular-thermodynamic model for the micellization of nonionic surfactants in aqueous solution.J Phys Chem B. 2007 Feb 8;111(5):1045-62. doi: 10.1021/jp065697a. J Phys Chem B. 2007. PMID: 17266258
-
Complementary use of simulations and molecular-thermodynamic theory to model micellization.Langmuir. 2006 Feb 14;22(4):1500-13. doi: 10.1021/la052042c. Langmuir. 2006. PMID: 16460068 Review.
-
A Review on Interactions between Amino Acids and Surfactants as Well as Their Impact on Corrosion Inhibition.ACS Omega. 2022 Dec 16;7(51):47471-47489. doi: 10.1021/acsomega.2c03629. eCollection 2022 Dec 27. ACS Omega. 2022. PMID: 36591120 Free PMC article. Review.
Cited by
-
Microbially derived surfactants: an ecofriendly, innovative, and effective approach for managing environmental contaminants.Front Bioeng Biotechnol. 2024 Aug 26;12:1398210. doi: 10.3389/fbioe.2024.1398210. eCollection 2024. Front Bioeng Biotechnol. 2024. PMID: 39253704 Free PMC article. Review.
References
-
- Schramm LL, Stasiuk EN, Marangoni DG. 2 Surfactants and their applications. Ann. Rep. Sect. C (Phys. Chem.) 2003;99:3–48. doi: 10.1039/B208499F. - DOI
-
- Massarweh O, Abushaikha AS. The use of surfactants in enhanced oil recovery: A review of recent advances. Energy Rep. 2020;6:3150–3178. doi: 10.1016/j.egyr.2020.11.009. - DOI
-
- Suárez L, Díez MA, García R, Riera FA. Membrane technology for the recovery of detergent compounds: A review. J. Ind. Eng. Chem. 2012;18:1859–1873. doi: 10.1016/j.jiec.2012.05.015. - DOI
-
- Falbe, J. Surfactants in Consumer Products: Theory, Technology and Application. (Springer Science & Business Media, 2012).
-
- Hellgren A-C, Weissenborn P, Holmberg K. Surfactants in water-borne paints. Prog. Org. Coat. 1999;35:79–87. doi: 10.1016/S0300-9440(99)00013-2. - DOI
LinkOut - more resources
Full Text Sources