De novo detection of somatic mutations in high-throughput single-cell profiling data sets
- PMID: 37414936
- PMCID: PMC11098751
- DOI: 10.1038/s41587-023-01863-z
De novo detection of somatic mutations in high-throughput single-cell profiling data sets
Abstract
Characterization of somatic mutations at single-cell resolution is essential to study cancer evolution, clonal mosaicism and cell plasticity. Here, we describe SComatic, an algorithm designed for the detection of somatic mutations in single-cell transcriptomic and ATAC-seq (assay for transposase-accessible chromatin sequence) data sets directly without requiring matched bulk or single-cell DNA sequencing data. SComatic distinguishes somatic mutations from polymorphisms, RNA-editing events and artefacts using filters and statistical tests parameterized on non-neoplastic samples. Using >2.6 million single cells from 688 single-cell RNA-seq (scRNA-seq) and single-cell ATAC-seq (scATAC-seq) data sets spanning cancer and non-neoplastic samples, we show that SComatic detects mutations in single cells accurately, even in differentiated cells from polyclonal tissues that are not amenable to mutation detection using existing methods. Validated against matched genome sequencing and scRNA-seq data, SComatic achieves F1 scores between 0.6 and 0.7 across diverse data sets, in comparison to 0.2-0.4 for the second-best performing method. In summary, SComatic permits de novo mutational signature analysis, and the study of clonal heterogeneity and mutational burdens at single-cell resolution.
© 2023. The Author(s).
Conflict of interest statement
The authors declare no competing interests.
Figures
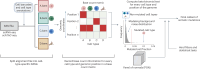
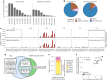
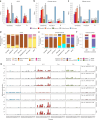
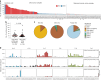
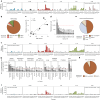
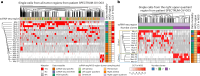
Similar articles
-
Benchmarking Algorithms for Gene Set Scoring of Single-cell ATAC-seq Data.Genomics Proteomics Bioinformatics. 2024 Jul 3;22(2):qzae014. doi: 10.1093/gpbjnl/qzae014. Genomics Proteomics Bioinformatics. 2024. PMID: 39049508 Free PMC article.
-
Hydrop enables droplet-based single-cell ATAC-seq and single-cell RNA-seq using dissolvable hydrogel beads.Elife. 2022 Feb 23;11:e73971. doi: 10.7554/eLife.73971. Elife. 2022. PMID: 35195064 Free PMC article.
-
LINEAGE: Label-free identification of endogenous informative single-cell mitochondrial RNA mutation for lineage analysis.Proc Natl Acad Sci U S A. 2022 Feb 1;119(5):e2119767119. doi: 10.1073/pnas.2119767119. Proc Natl Acad Sci U S A. 2022. PMID: 35086932 Free PMC article.
-
Application of Single-Cell Assay for Transposase-Accessible Chromatin with High Throughput Sequencing in Plant Science: Advances, Technical Challenges, and Prospects.Int J Mol Sci. 2024 Jan 25;25(3):1479. doi: 10.3390/ijms25031479. Int J Mol Sci. 2024. PMID: 38338756 Free PMC article. Review.
-
Mitochondrial single-cell ATAC-seq for high-throughput multi-omic detection of mitochondrial genotypes and chromatin accessibility.Nat Protoc. 2023 May;18(5):1416-1440. doi: 10.1038/s41596-022-00795-3. Epub 2023 Feb 15. Nat Protoc. 2023. PMID: 36792778 Free PMC article. Review.
Cited by
-
Recent Advances in Genomic Approaches for the Detection of Homologous Recombination Deficiency.Cancer Res Treat. 2024 Oct;56(4):975-990. doi: 10.4143/crt.2024.154. Epub 2024 Jul 17. Cancer Res Treat. 2024. PMID: 39026430 Free PMC article. Review.
-
Sex as a biological variable in ageing: insights and perspectives on the molecular and cellular hallmarks.Open Biol. 2024 Oct;14(10):240177. doi: 10.1098/rsob.240177. Epub 2024 Oct 30. Open Biol. 2024. PMID: 39471841 Free PMC article. Review.
-
Refining SARS-CoV-2 intra-host variation by leveraging large-scale sequencing data.NAR Genom Bioinform. 2024 Nov 12;6(4):lqae145. doi: 10.1093/nargab/lqae145. eCollection 2024 Sep. NAR Genom Bioinform. 2024. PMID: 39534500 Free PMC article.
-
Tissue-Predisposition to Cancer Driver Mutations.Cells. 2024 Jan 5;13(2):106. doi: 10.3390/cells13020106. Cells. 2024. PMID: 38247798 Free PMC article. Review.
-
Phertilizer: Growing a clonal tree from ultra-low coverage single-cell DNA sequencing of tumors.PLoS Comput Biol. 2023 Oct 11;19(10):e1011544. doi: 10.1371/journal.pcbi.1011544. eCollection 2023 Oct. PLoS Comput Biol. 2023. PMID: 37819942 Free PMC article.
References
MeSH terms
Grants and funding
LinkOut - more resources
Full Text Sources