Implementing Precision Psychiatry: A Systematic Review of Individualized Prediction Models for Clinical Practice
- PMID: 32914178
- PMCID: PMC7965077
- DOI: 10.1093/schbul/sbaa120
Implementing Precision Psychiatry: A Systematic Review of Individualized Prediction Models for Clinical Practice
Abstract
Background: The impact of precision psychiatry for clinical practice has not been systematically appraised. This study aims to provide a comprehensive review of validated prediction models to estimate the individual risk of being affected with a condition (diagnostic), developing outcomes (prognostic), or responding to treatments (predictive) in mental disorders.
Methods: PRISMA/RIGHT/CHARMS-compliant systematic review of the Web of Science, Cochrane Central Register of Reviews, and Ovid/PsycINFO databases from inception until July 21, 2019 (PROSPERO CRD42019155713) to identify diagnostic/prognostic/predictive prediction studies that reported individualized estimates in psychiatry and that were internally or externally validated or implemented. Random effect meta-regression analyses addressed the impact of several factors on the accuracy of prediction models.
Findings: Literature search identified 584 prediction modeling studies, of which 89 were included. 10.4% of the total studies included prediction models internally validated (n = 61), 4.6% models externally validated (n = 27), and 0.2% (n = 1) models considered for implementation. Across validated prediction modeling studies (n = 88), 18.2% were diagnostic, 68.2% prognostic, and 13.6% predictive. The most frequently investigated condition was psychosis (36.4%), and the most frequently employed predictors clinical (69.5%). Unimodal compared to multimodal models (β = .29, P = .03) and diagnostic compared to prognostic (β = .84, p < .0001) and predictive (β = .87, P = .002) models were associated with increased accuracy.
Interpretation: To date, several validated prediction models are available to support the diagnosis and prognosis of psychiatric conditions, in particular, psychosis, or to predict treatment response. Advancements of knowledge are limited by the lack of implementation research in real-world clinical practice. A new generation of implementation research is required to address this translational gap.
Keywords: evidence; implementation; individualized; prediction; prevention; prognosis; risk; validation.
© The Author(s) 2020. Published by Oxford University Press on behalf of the Maryland Psychiatric Research Center.
Figures
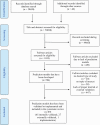
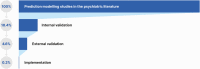
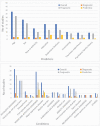
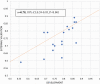
Similar articles
-
Clinical prediction models in psychiatry: a systematic review of two decades of progress and challenges.Mol Psychiatry. 2022 Jun;27(6):2700-2708. doi: 10.1038/s41380-022-01528-4. Epub 2022 Apr 1. Mol Psychiatry. 2022. PMID: 35365801 Free PMC article.
-
Beyond the black stump: rapid reviews of health research issues affecting regional, rural and remote Australia.Med J Aust. 2020 Dec;213 Suppl 11:S3-S32.e1. doi: 10.5694/mja2.50881. Med J Aust. 2020. PMID: 33314144
-
Using Electronic Health Records to Facilitate Precision Psychiatry.Biol Psychiatry. 2024 Oct 1;96(7):532-542. doi: 10.1016/j.biopsych.2024.02.1006. Epub 2024 Feb 24. Biol Psychiatry. 2024. PMID: 38408535 Review.
-
Assessing prognosis and prediction of treatment response in early rheumatoid arthritis: systematic reviews.Health Technol Assess. 2018 Nov;22(66):1-294. doi: 10.3310/hta22660. Health Technol Assess. 2018. PMID: 30501821 Free PMC article.
-
Real-World Implementation of Precision Psychiatry: A Systematic Review of Barriers and Facilitators.Brain Sci. 2022 Jul 16;12(7):934. doi: 10.3390/brainsci12070934. Brain Sci. 2022. PMID: 35884740 Free PMC article. Review.
Cited by
-
Identifying adolescents at risk for depression: Assessment of a global prediction model in the Great Smoky Mountains Study.J Psychiatr Res. 2022 Nov;155:146-152. doi: 10.1016/j.jpsychires.2022.08.017. Epub 2022 Aug 20. J Psychiatr Res. 2022. PMID: 36029626 Free PMC article.
-
Development and validation of a prognostic model for the early identification of COVID-19 patients at risk of developing common long COVID symptoms.Diagn Progn Res. 2022 Nov 17;6(1):22. doi: 10.1186/s41512-022-00135-9. Diagn Progn Res. 2022. PMID: 36384641 Free PMC article.
-
The importance of external validation to advance precision psychiatry.Lancet Reg Health Eur. 2022 Aug 26;22:100498. doi: 10.1016/j.lanepe.2022.100498. eCollection 2022 Nov. Lancet Reg Health Eur. 2022. PMID: 36061494 Free PMC article. No abstract available.
-
Development and Validation of Predictive Model for a Diagnosis of First Episode Psychosis Using the Multinational EU-GEI Case-control Study and Modern Statistical Learning Methods.Schizophr Bull Open. 2023 Mar 10;4(1):sgad008. doi: 10.1093/schizbullopen/sgad008. eCollection 2023 Jan. Schizophr Bull Open. 2023. PMID: 39145333 Free PMC article.
-
Electronic health record-based prediction models for in-hospital adverse drug event diagnosis or prognosis: a systematic review.J Am Med Inform Assoc. 2023 Apr 19;30(5):978-988. doi: 10.1093/jamia/ocad014. J Am Med Inform Assoc. 2023. PMID: 36805926 Free PMC article.
References
-
- Genetics Reference. What is precision medicine?https://ghr.nlm.nih.gov/primer/precisionmedicine/definition. Accessed March 1, 2020.
-
- Fusar-Poli P, Hijazi Z, Stahl D, Steyerberg EW. The science of prognosis in psychiatry: a review. JAMA Psychiatry. 2018;75(12):1289–1297. - PubMed
-
- Wolff RF, Moons KGM, Riley RD, et al. ; PROBAST Group . PROBAST: a tool to assess the risk of bias and applicability of prediction model studies. Ann Intern Med. 2019;170(1):51–58. - PubMed
Publication types
MeSH terms
Grants and funding
LinkOut - more resources
Full Text Sources
Other Literature Sources
Medical
Miscellaneous