Semi-automated screening of biomedical citations for systematic reviews
- PMID: 20102628
- PMCID: PMC2824679
- DOI: 10.1186/1471-2105-11-55
Semi-automated screening of biomedical citations for systematic reviews
Abstract
Background: Systematic reviews address a specific clinical question by unbiasedly assessing and analyzing the pertinent literature. Citation screening is a time-consuming and critical step in systematic reviews. Typically, reviewers must evaluate thousands of citations to identify articles eligible for a given review. We explore the application of machine learning techniques to semi-automate citation screening, thereby reducing the reviewers' workload.
Results: We present a novel online classification strategy for citation screening to automatically discriminate "relevant" from "irrelevant" citations. We use an ensemble of Support Vector Machines (SVMs) built over different feature-spaces (e.g., abstract and title text), and trained interactively by the reviewer(s). Semi-automating the citation screening process is difficult because any such strategy must identify all citations eligible for the systematic review. This requirement is made harder still due to class imbalance; there are far fewer "relevant" than "irrelevant" citations for any given systematic review. To address these challenges we employ a custom active-learning strategy developed specifically for imbalanced datasets. Further, we introduce a novel undersampling technique. We provide experimental results over three real-world systematic review datasets, and demonstrate that our algorithm is able to reduce the number of citations that must be screened manually by nearly half in two of these, and by around 40% in the third, without excluding any of the citations eligible for the systematic review.
Conclusions: We have developed a semi-automated citation screening algorithm for systematic reviews that has the potential to substantially reduce the number of citations reviewers have to manually screen, without compromising the quality and comprehensiveness of the review.
Figures
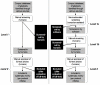
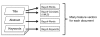
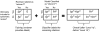
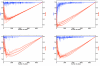
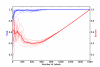
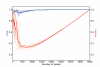
Similar articles
-
Faster title and abstract screening? Evaluating Abstrackr, a semi-automated online screening program for systematic reviewers.Syst Rev. 2015 Jun 15;4:80. doi: 10.1186/s13643-015-0067-6. Syst Rev. 2015. PMID: 26073974 Free PMC article.
-
A semi-supervised approach using label propagation to support citation screening.J Biomed Inform. 2017 Aug;72:67-76. doi: 10.1016/j.jbi.2017.06.018. Epub 2017 Jun 23. J Biomed Inform. 2017. PMID: 28648605 Free PMC article.
-
Topic detection using paragraph vectors to support active learning in systematic reviews.J Biomed Inform. 2016 Aug;62:59-65. doi: 10.1016/j.jbi.2016.06.001. Epub 2016 Jun 10. J Biomed Inform. 2016. PMID: 27293211 Free PMC article.
-
Expediting citation screening using PICo-based title-only screening for identifying studies in scoping searches and rapid reviews.Syst Rev. 2017 Nov 25;6(1):233. doi: 10.1186/s13643-017-0629-x. Syst Rev. 2017. PMID: 29178925 Free PMC article. Review.
-
Machine Learning Methods in Systematic Reviews: Identifying Quality Improvement Intervention Evaluations [Internet].Rockville (MD): Agency for Healthcare Research and Quality (US); 2012 Sep. Report No.: 12-EHC125-EF. Rockville (MD): Agency for Healthcare Research and Quality (US); 2012 Sep. Report No.: 12-EHC125-EF. PMID: 23101052 Free Books & Documents. Review.
Cited by
-
Efficiency and Workload Reduction of Semi-automated Citation Screening Software for Creating Clinical Practice Guidelines: A Prospective Observational Study.J Epidemiol. 2024 Aug 5;34(8):380-386. doi: 10.2188/jea.JE20230227. Epub 2024 May 31. J Epidemiol. 2024. PMID: 38105001 Free PMC article.
-
Rapid network meta-analysis using data from Food and Drug Administration approval packages is feasible but with limitations.J Clin Epidemiol. 2019 Oct;114:84-94. doi: 10.1016/j.jclinepi.2019.06.010. Epub 2019 Jun 18. J Clin Epidemiol. 2019. PMID: 31226413 Free PMC article.
-
Adverse events following implantable cardioverter defibrillator implantation: a systematic review.J Interv Card Electrophysiol. 2014 Aug;40(2):191-205. doi: 10.1007/s10840-014-9913-z. Epub 2014 Jun 20. J Interv Card Electrophysiol. 2014. PMID: 24948126 Review.
-
Making Science Computable Using Evidence-Based Medicine on Fast Healthcare Interoperability Resources: Standards Development Project.J Med Internet Res. 2024 Jun 25;26:e54265. doi: 10.2196/54265. J Med Internet Res. 2024. PMID: 38916936 Free PMC article.
-
Using text mining for study identification in systematic reviews: a systematic review of current approaches.Syst Rev. 2015 Jan 14;4(1):5. doi: 10.1186/2046-4053-4-5. Syst Rev. 2015. PMID: 25588314 Free PMC article. Review.
References
-
- Counsell C. Formulating questions and locating primary studies for inclusion in systematic reviews. Ann Intern Med. 1997;127:380–387. - PubMed
-
- Wheeler P, Balk E, Bresnahan K, Shephard B, Lau J, DeVine D, Chung M, Miller K. Criteria for determining disability in infants and children: short stature. Evidence Report/Technology Assessment No. 73. Prepared by New England Medical Center Evidence-based Practice Center under Contract No. 290-97-001. 2003. - PMC - PubMed
-
- Cole C, Binney G, Casey P, Fiascone J, Hagadorn J, Kim C, Wang C, Devine D, Miller K, Lau J. Criteria for determining disability in infants and children: Low Birth Weight. Evidence Report/Technology Assessment No. 70. Prepared by New England Medical Center Evidence-based Practice Center under Contract No. 290-97-0019. 2002. - PMC - PubMed
-
- Perrin E, Cole C, Frank D, Glicken S, Guerina N, Petit K, Sege R, Volpe M, Chew P, MeFadden C, Devine D, Miller K, Lau J. Criteria for determining disability in infants and children: failure to thrive. Evidence Report/Technology Assessment No. 72. Prepared by New England Medical Center Evidence-based Practice Center under Contract No. 290-97-0019. 2003. - PMC - PubMed
Publication types
MeSH terms
Grants and funding
LinkOut - more resources
Full Text Sources